In today’s digital age, the integration of Artificial Intelligence (AI) into various aspects of our lives has become increasingly prevalent. However, as AI systems continue to evolve, the issue of bias and fairness in algorithmic decision-making has garnered significant attention. As an expert in the field, I delve into the complex realm of Ethical AI, exploring the challenges and solutions to ensure equitable outcomes.
Understanding Ethical AI
The Importance of Ethics in AI
- Ensuring ethical practices within Artificial Intelligence (AI) is crucial in today’s digital landscape. Ethical AI involves upholding values that prioritize fairness, transparency, and accountability in algorithmic decision-making processes. It’s essential to establish ethical guidelines to safeguard against biases that can lead to discriminatory outcomes. By integrating ethical considerations into AI development, we can foster trust and confidence in these technologies.
Defining Bias and Fairness in Algorithms
- Bias in algorithms refers to the skewing of results or decisions in a systematic and unfair manner. Recognizing and mitigating bias is vital to maintain fairness in algorithmic systems. Fairness, on the other hand, involves ensuring that AI algorithms are impartial and do not discriminate against individuals based on protected characteristics such as race, gender, or ethnicity. Striving for fairness in AI is essential to building inclusive and equitable digital environments.
Key Challenges in Achieving Ethical AI
Recognizing Invisible Biases
- In AI systems, identifying covert biases is one of the primary obstacles in ensuring fairness. These hidden prejudices may stem from historical data patterns, societal norms, or even unintentional coding errors. Detecting these unseen biases requires meticulous scrutiny of the algorithms’ outcomes, as well as continuous monitoring for discriminatory results. By acknowledging and uncovering these implicit biases, developers can take remedial actions to rectify the unfair treatment of individuals or groups within algorithmic systems.
The Complexity of Measuring Fairness
- Measuring fairness in AI algorithms is a multifaceted task due to the various interpretations of what constitutes fairness. Evaluating fairness requires a nuanced approach that considers not only statistical parity but also the impacts on diverse demographics. Determining a universal metric for fairness is challenging, as different stakeholders may prioritize distinct fairness criteria based on their perspectives and goals. Balancing these competing notions of fairness while designing and evaluating AI systems poses a significant challenge in ensuring equitable outcomes for all users.
Frameworks and Principles for Ethical AI
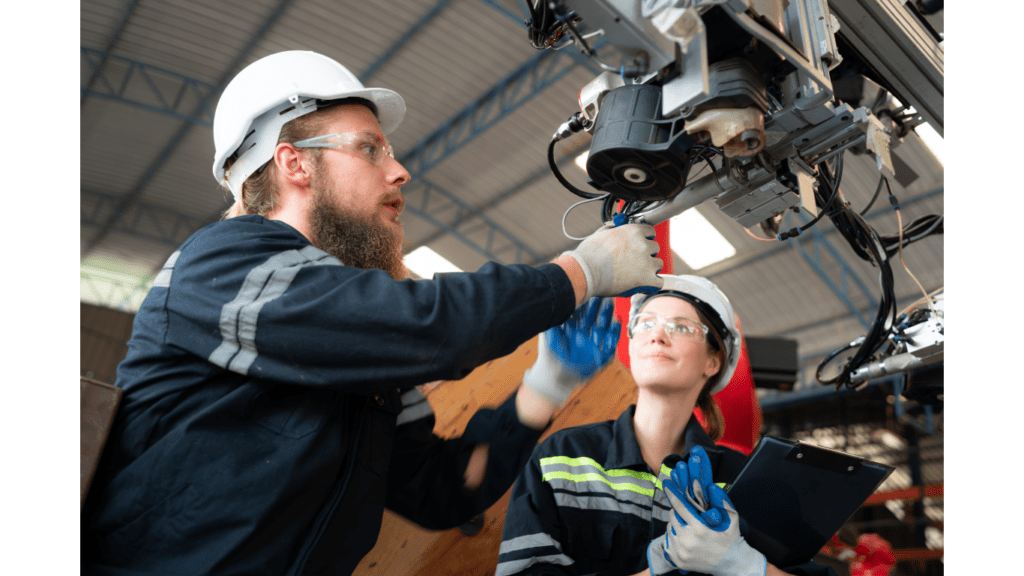
1. Existing Ethical Guidelines for AI Development
In the realm of AI development, adhering to established ethical guidelines is paramount to ensure the responsible and unbiased deployment of algorithmic systems. Ethical frameworks such as the IEEE’s Global Initiative for Ethical Considerations in Artificial Intelligence and Autonomous Systems provide valuable principles for developers like me to follow. These guidelines emphasize the significance of transparency, accountability, and the protection of user privacy in AI applications. By incorporating these existing ethical standards, I can contribute to the creation of AI systems that prioritize fairness and uphold ethical values.2. Implementing Principles in Algorithm Design
When it comes to designing algorithms, integrating ethical principles is a fundamental step in promoting fairness and mitigating bias. As I engage in algorithm design, I am committed to incorporating principles that prioritize fairness and equity for all users. Concepts like fairness, accountability, and explainability are essential considerations in algorithm development to ensure that the systems I create do not perpetuate discriminatory outcomes. By implementing these principles proactively, I can contribute to the advancement of AI technologies that align with ethical standards and promote equitable treatment for diverse user populations.Case Studies: Ethical AI in Action
Success Stories in Mitigating Bias
- In exploring successful efforts to combat bias in AI systems, I delve into a notable case where a leading tech company implemented bias mitigation strategies effectively. By utilizing diverse datasets that reflect the demographic variety of their user base, the company was able to train its algorithms to recognize and neutralize biases. Through continuous monitoring and adjustment, they achieved more equitable outcomes for all users. This case study illustrates the importance of proactive measures in ensuring algorithmic fairness.
Lessons Learned from Failures
- Reflecting on failures in addressing bias in AI, I examine a case study where an e-commerce platform faced backlash due to biased recommendations leading to discriminatory outcomes. The platform had overlooked the diverse needs of its user base, resulting in algorithmic decisions that favored certain groups over others. This failure highlighted the critical importance of comprehensive testing across varied demographic segments to uncover and rectify biases. Lessons from such failures emphasize the necessity of inclusivity and constant vigilance in combating bias within AI systems.
The Role of Regulation in Ensuring Ethical AI
Government Policies Shaping AI Development
In discussing the role of regulation in ensuring Ethical AI, I recognize the crucial impact of government policies on shaping AI development. Government bodies play a vital role in setting the standards and guidelines that AI developers and organizations must adhere to. By implementing clear regulations, governments can promote Ethical AI practices that prioritize fairness, transparency, and accountability in algorithmic systems. These policies help in mitigating biases and ensuring that AI technologies are developed and used responsibly.Global Standards for AI Fairness
When considering global standards for AI fairness, I emphasize the importance of creating a unified framework that transcends geographical boundaries. Establishing universal guidelines for AI fairness helps in creating a level playing field for all users and stakeholders worldwide. By harmonizing standards across countries and regions, we can work towards a more ethical and inclusive AI ecosystem. Collaboration on a global scale is essential to address bias and promote fairness in algorithmic systems, ensuring that AI technologies benefit society as a whole.Tools and Techniques to Address Bias
Utilizing AI Audits and Impact Assessments
In addressing bias in AI systems, conducting AI audits and impact assessments is crucial. These processes involve evaluating the algorithms, data inputs, and outcomes to identify and mitigate potential biases. It’s essential to regularly audit AI systems to ensure fairness and accountability in decision-making processes. By assessing the impact of AI on different demographic groups, organizations can proactively address biases and make necessary adjustments.Innovations in Algorithmic Bias Detection
Innovations in algorithmic bias detection are becoming increasingly important in the development of Ethical AI. Techniques such as algorithmic impact assessments and fairness indicators help in detecting and quantifying biases within AI models. Leveraging advanced technologies like machine learning algorithms, researchers can uncover hidden biases and work towards creating more transparent and fair AI systems. Continuous advancements in bias detection methods are essential for enhancing algorithmic fairness and promoting ethical practices in AI development.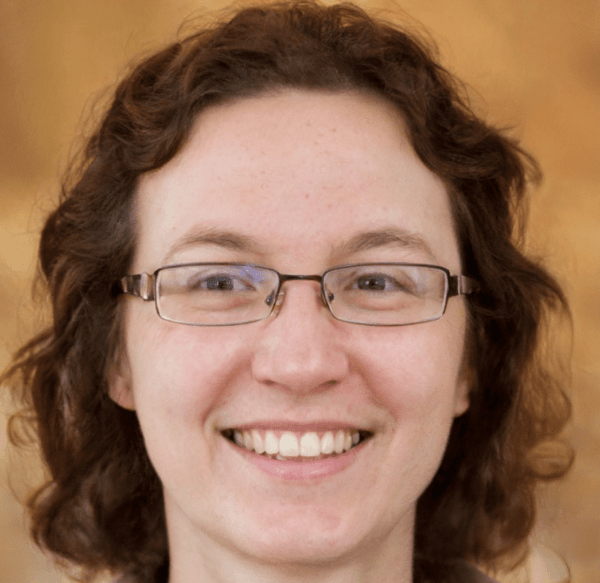
About the author:
Joanna Smith
Joanna Smith is a distinguished journalist who has made a significant impact at Whisper Wagon Wire, a premier platform renowned for its exclusive insights into top stories, world news, science, technology, and home trends. With a natural talent for storytelling and a dedication to uncovering the truth, Joanna has built a remarkable career in journalism. Joanna’s journey began with a strong educational foundation in journalism and communication.